Fluid Intelligence: Understanding Liquid Neural Networks
- Nagesh Singh Chauhan
- Dec 20, 2024
- 4 min read
Adaptive Intelligence for Dynamic Environments
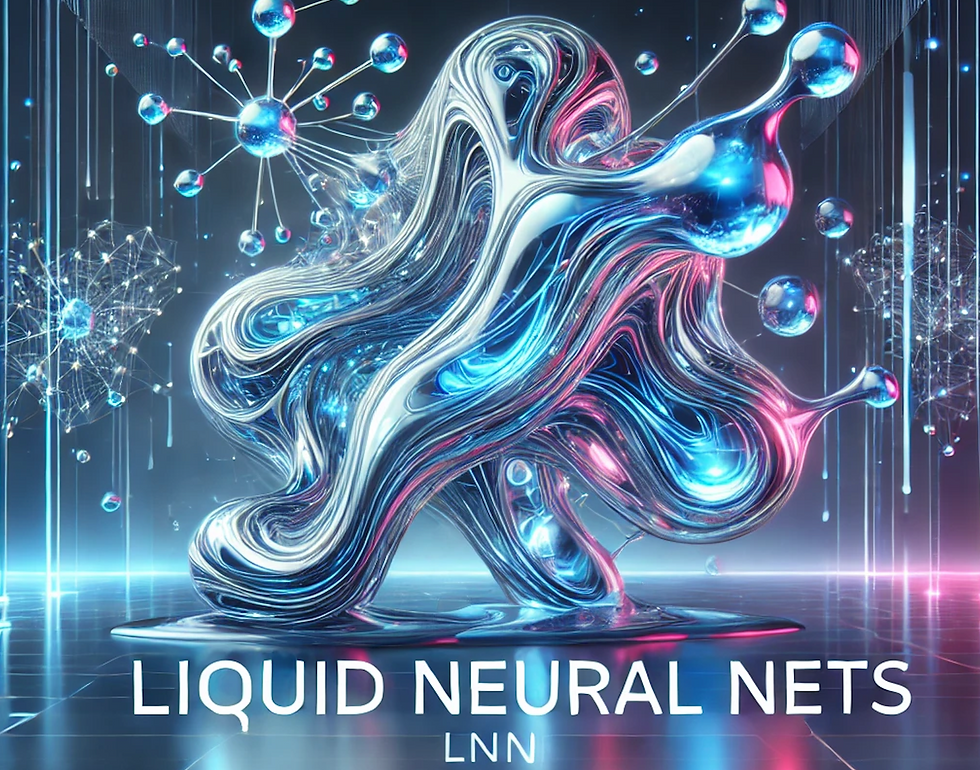
Introduction
In the ever-evolving landscape of artificial intelligence, a revolutionary approach to neural networks has emerged: Liquid Neural Networks (LNNs). These innovative systems represent a significant leap forward in how we think about and implement machine learning, offering unique advantages over traditional neural networks. Let's dive deep into what makes LNNs special and why they might shape the future of AI.
The Need for Liquid Neural Networks in Modern Deep Learning
Deep learning has revolutionized artificial intelligence, pushing the boundaries of what machines can achieve. However, as we venture deeper into more complex applications, traditional deep neural networks are revealing their limitations. These challenges have sparked the need for more adaptive and efficient solutions, leading to the emergence of Liquid Neural Networks (LNNs).
Current Challenges in Deep Learning
Traditional neural networks face several critical limitations:
Resource Intensity
Require massive computational infrastructure
Need extensive datasets for effective training
Consume significant energy during training and inference
Demand substantial memory resources for model parameters
Architectural Rigidity
Once trained, networks maintain fixed architectures
Struggle to adapt to new, unseen situations
Poor performance on out-of-distribution data
Limited ability to handle dynamic changes in input patterns
The black-box nature of traditional neural networks also poses significant challenges for industries where transparency and interpretability are crucial. This lack of transparency makes it difficult to trust and validate their decisions, especially in sensitive applications like healthcare and autonomous systems.
Nature's Blueprint: The Inspiration Behind LNNs
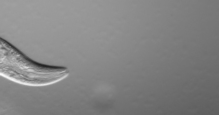
At the heart of this innovation lies an unlikely muse: a microscopic worm known as C. elegans. This remarkable creature, with merely 302 neurons, exhibits complex behaviors that seem to transcend its simple neural architecture. Like a master artist creating masterpieces with a limited palette, C. elegans demonstrates that neural efficiency matters more than neural quantity. This revelation sparked a profound question: Could we create artificial neural networks that mirror this biological elegance?
The Liquid Revolution
Imagine water flowing through channels, adapting seamlessly to any container's shape while maintaining its essential properties. This fluid adaptability forms the core metaphor for Liquid Neural Networks. Unlike traditional neural networks, which operate within rigid, predetermined structures, LNNs flow and adapt, reshaping their computational pathways in response to new information.
Architecture of Fluidity
The architecture of an LNN unfolds across three distinctive layers, each playing a crucial role in the network's dynamic behavior:
The Input Gateway
Serves as the initial contact point for data
Transforms raw information into processable signals
Prepares data for the dynamic liquid layer
The Liquid Core (Reservoir)
Houses a sophisticated network of interconnected neurons
Maintains initialized synaptic weights that evolve over time
Creates a rich, dynamic environment for information processing
The Output Horizon
Synthesizes processed information
Translates complex patterns into meaningful outputs
Maintains the network's interpretability
The Dance of Dynamics
At the heart of LNNs lies a mathematical ballet of first-order dynamical systems. These systems, controlled by non-linear interlinked gates, create a living, breathing network that evolves with each new input. Time constants shift and adapt, hidden states transform, and the network learns not just from data, but from its own dynamic interactions.
Beyond Traditional Boundaries
What truly sets LNNs apart is their biological plausibility. While traditional networks march forward in discrete, digital steps, LNNs flow through continuous differential equations, mirroring the smooth, analog nature of biological neural processing. This approach yields several remarkable advantages:
Adaptive Intelligence
Dynamic structure modification based on input patterns
Continuous learning capability post-initial training
Sophisticated handling of temporal dependencies
Seamless adaptation to novel scenarios
Computational Elegance
Streamlined parameter requirements
Reduced training data dependency
Optimized resource utilization
Enhanced performance with minimalist models
Key Advantages of Liquid Neural Networks
1. Adaptive Learning
- Can learn on the job even after initial training
- Dynamically adjusts to new situations and data patterns
- More flexible than traditional neural networks
2. Efficiency
- Requires less computational resources
- Needs smaller training datasets
- Produces less model complexity
3. Processing Capabilities
- Excellent at handling time-series data
- Can process multi-modal information from different time scales
- Better at mimicking biological brain functions
4. Scalability
- More scalable at the enterprise level
- Requires less labeled data
- Better suited for real-world applications
Real-World Applications
LNNs have shown promising results in various fields:
Autonomous Systems
- Drones: LNN-powered drones have shown superior performance in unknown territories
- Self-Driving Cars: Better adaptation to unexpected situations
- Robotics: Improved handling of unpredictable environmental interactions
Medical Applications
- Medical image analysis
- Health records processing
- Biomedical signal analysis
- Real-time diagnostics
Natural Language Processing
- More efficient processing of large text datasets
- Better handling of sentiment analysis
- Improved entity recognition
Challenges and Limitations
Despite their advantages, LNNs face several challenges:
1. Vanishing Gradient Problem: Similar to traditional neural networks
2. Hyperparameter Tuning: Complex due to randomness in the liquid layer
3. Limited Resources: Still a relatively new technology with fewer implementation resources
4. Processing Speed: Can be slow in real-world scenarios
5. Data Requirements: Works best with time-series data, less effective with regular tabular data
Future Prospects
The future of LNNs looks promising, particularly in areas requiring adaptive learning and real-time processing. Recent implementations on neuromorphic hardware have shown impressive results, with accuracy rates reaching 91.3% while maintaining energy efficiency. This suggests that LNNs could play a crucial role in the next generation of AI systems, particularly in edge computing and IoT applications.
Conclusion
Liquid Neural Networks represent a significant advancement in neural network architecture, offering a more flexible and efficient approach to machine learning. While they face certain challenges, their unique advantages make them particularly well-suited for applications requiring adaptive learning and real-time processing. As research continues and implementation resources grow, we can expect to see LNNs playing an increasingly important role in shaping the future of artificial intelligence.
The combination of biological inspiration and innovative engineering in LNNs points to a future where AI systems can be more adaptive, efficient, and capable of handling complex real-world scenarios. As we continue to push the boundaries of what's possible in AI, Liquid Neural Networks stand as a testament to how mimicking nature's solutions can lead to breakthrough innovations in technology.
Comments